Prof Denis Leung
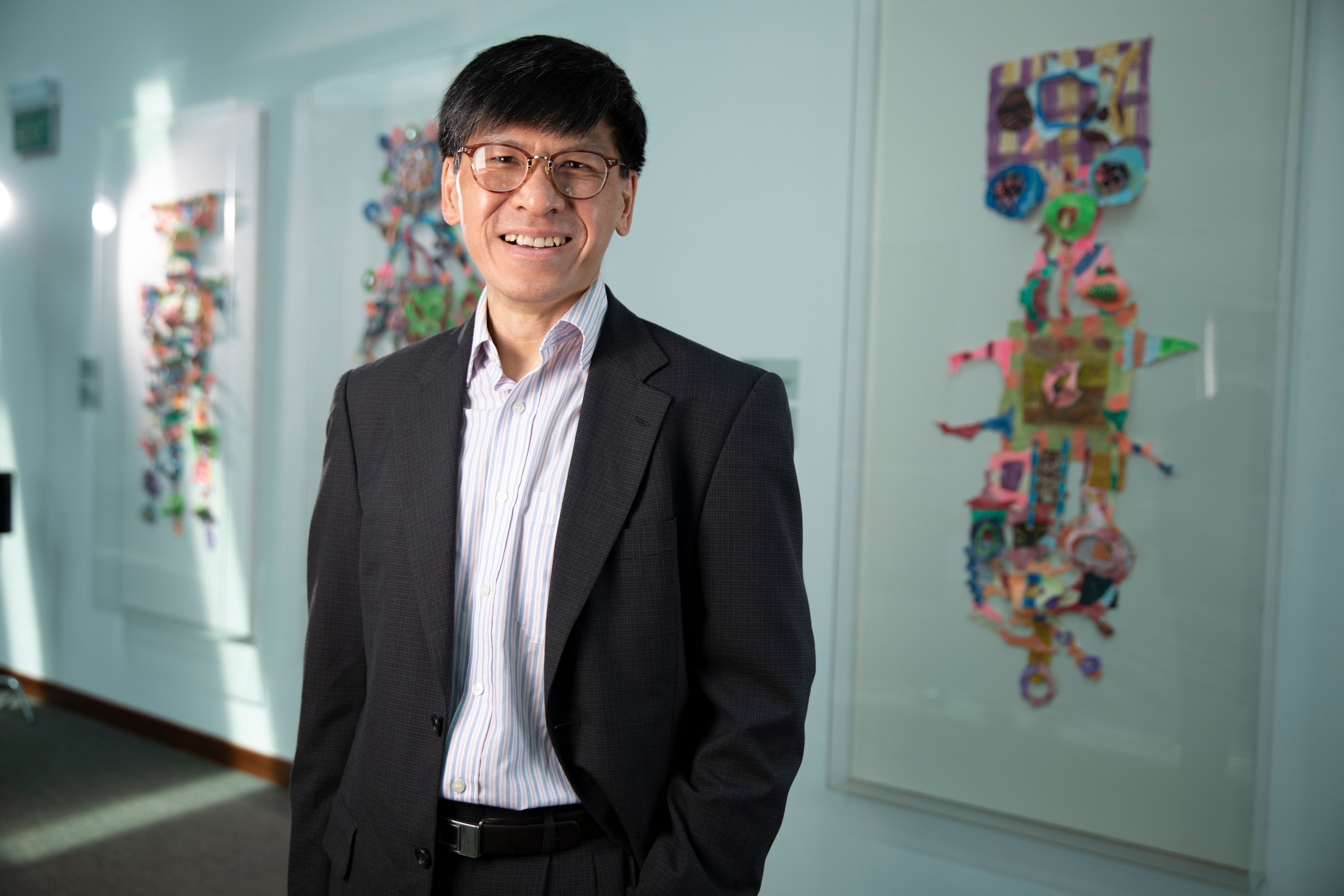
Feature on Prof Denis Leung, Professor of Statistics
School of Economics, Singapore Management University
Making sense of data to make life better
Professor Denis Leung’s ability to plug data gaps and unlock the mysteries of patient data has led to more personalised treatments and the delivery new drugs with fewer clinical trials.
Over the last three decades, the speed and amount of data collected has grown exponentially. Playing a pivotal role in this ever-important space is Professor Denis Leung, who has been working alongside the researchers collecting the data, helping them to turn information into meaningful findings to improve systems and lives.
“For a long time, statisticians struggled with collecting sufficient data for them to work with,” says Prof Leung. “The big challenge was making sense of incomplete data: paper-based surveys and our reluctance to give precise answers about personal things like age and income left gaps for statisticians to replace with credible and trusted estimates.”
This is where Prof Leung’s 30 years of experience comes in, devising new tools to analyse data, or somewhat paradoxically, missing data, which he has done since his first day of work at the Chinese University of Hong Kong’s Faculty of Medicine.
“My first job was with clinicians and researchers, making sense of patient data and treatment outcomes. Why did some patients get better while others not? Why did some treatments work in one way but not in other ways?” says Prof Leung, who continued this work in New York for almost ten years where he applied his expertise in oncology and carcinogenesis.
He worked in a hospital with scientists and clinicians who subsequently became life-long research collaborators. “We worked very well together to solve problems for patients with severe diseases. Every week we'd get together—surgeons, pathologists, oncologists, basic scientists, statisticians, data scientists—to look at patient data and design patient management pathways,” he says. “These pathways allow clinicians to advise on appropriate treatment options, and also let patients and their families make informed decisions.”
According to Prof Leung, there's a certain point that any treatment will only make things worse. “Sometimes, you would rather see the patient spending more time with the family and living life rather than going for ineffective and toxic treatments—this is the very issue that has kept me motivated to do more,” he shares.
Indeed, he kept this very motivation when he moved to Singapore and SMU in 2001. He has since made significant contributions to the field of biomedicine and in 2020, a Stanford University report on the world’s most-cited researchers ranked Prof Leung among the top two percent of scientists in the field of oncology and carcinogenesis.
“Every problem in practice and data set has its unique characteristics. You often find that many issues cannot be comprehensively solved using existing methods,” he says. “Often, current knowledge is adapted to create new methods for addressing these specific challenges to provide better solutions.”
His time at SMU can be characterised as one of continual discovery of new statistical methods to problems and opportunities. In 2001, Controlled Clinical Trials 22(2) 126-138, Prof Leung developed a statistical rule to define a novel way of conducting phase one clinical trials. Traditionally, new drugs are first piloted with a small number of people to understand the drug’s adverse impacts. To expose fewer patients to these risks, he devised a rule which achieves the same degree of credibility but with fewer trial participants needed using conventional methods.
Keeping people at the heart of the digital age
Prof Leung remains deeply passionate about working on problems which would help people. His early experience in cross-disciplinary collaboration features prominently in his thoughts about how statisticians should adapt in the data age. Statistical methods, he believes, have an increasingly important and complementary role to play in uncovering the relationships and reasons behind conclusions generated through machine learning and artificial intelligence (AI).
“While algorithms can process massive amounts of data to derive a specific outcome such as diagnosing whether a person has a certain condition, they are unable to explain the mechanics or the ‘why’ behind this assessment,” he explains. “This is where statisticians come in—to determine the specific reasons behind the problem or the human element involved.”
Going forward, Prof Leung hopes that statisticians and computer scientists will collaborate deeply and learn from each other. “By combining the explanatory strength of statistics and the speed and predictive power of machine learning and AI algorithms, valuable insights can be derived to solve many complex real-world problems,” he says.