XIA YING
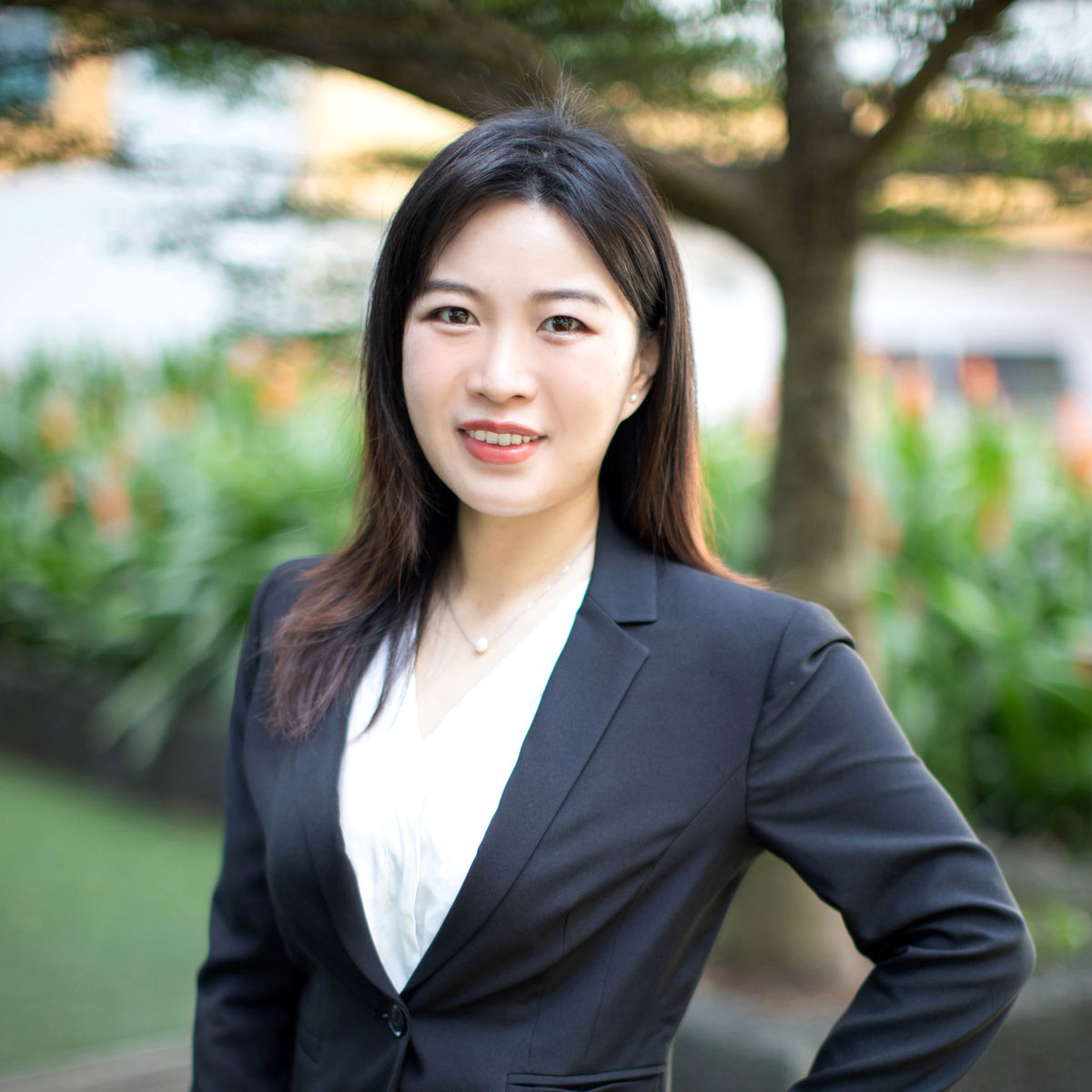
XIA YING
Ph.D Candidate in Economics
I am a job market candidate and I will be available for interviews.
REFERENCES
Professor SU Liangjun
Email: sulj@sem.tsinghua.edu.cn
Tel: +86 10 62789506
Professor Yichong ZHANG
Email: yczhang@smu.edu.sg
Tel: +65 68280881
Professor Peter C. B. PHILLIPS
Email: peter.phillips@yale.edu
Tel: (203) 432-3695
WORKING PAPERS
"Efficient Nonparametric Estimation of the Generalized Panel Data Transformation Models with Fixed Effects", (Job Market Paper)
In this article, we consider a generalized panel data transformation model with fixed effects where the structural functions are assumed to be additive. Our model does not impose parametric assumptions on the transformation function, the structural function, or the distribution of the idiosyncratic error term. We propose a multiple-stage Local Maximum Likelihood Estimator (LMLE) for the structural functions. In the first stage, we apply the regularized logistic sieve method to estimate the sieve coefficients associated with the approximation of a composite function and then apply a matching method to obtain initial consistent estimators of the additive structural functions. In the second stage, we apply the local polynomial method to estimate certain composite function and its derivatives to be used later on. In the third stage we apply the local linear method to obtain the refined estimator of the additive structural functions based on the estimators obtained in Steps 1 and 2. The greatest advantage is that all minimization problems are convex and thus overcome the computational hurdle for existing approaches to the generalized panel data transformation model. The final estimates of the additive terms achieve the optimal one-dimensional convergence rate, asymptotic normality and oracle efficiency. The Monte Carlo simulations demonstrate that our new estimator performs well in finite samples.
"Efficient Nonparametric Estimation of the Generalized Additive Model with an Unknown Link Function"
In this article, we consider a generalized additive model with an unknown link function (GAMULF). Our model does not impose parametric assumptions on the link function or the distribution of the idiosyncratic error term. We propose a three-stage nonparametric least squares (NPLS) estimation procedure for the additive functions. In the first stage, we estimate conditional expectation by the local-linear kernel regression and then apply matching method to the splines series to obtain initial estimators. In the second stage, we use the local-polynomial kernel regression to estimate the link function. In the third stage, given the estimators in Stages 1 and 2, we apply the local linear kernel regression to refine the initial estimator. The great advantage of such a procedure is that the estimators obtained at all stages have closed-form expressions, which overcomes the computational hurdle for existing estimators of the GAMULF model. The final estimators of the additive terms achieve the optimal one-dimensional convergence rate, asymptotic normality and oracle efficiency. Monte Carlo simulations demonstrate that our new estimator performs well in finite samples.