Wang Kexin
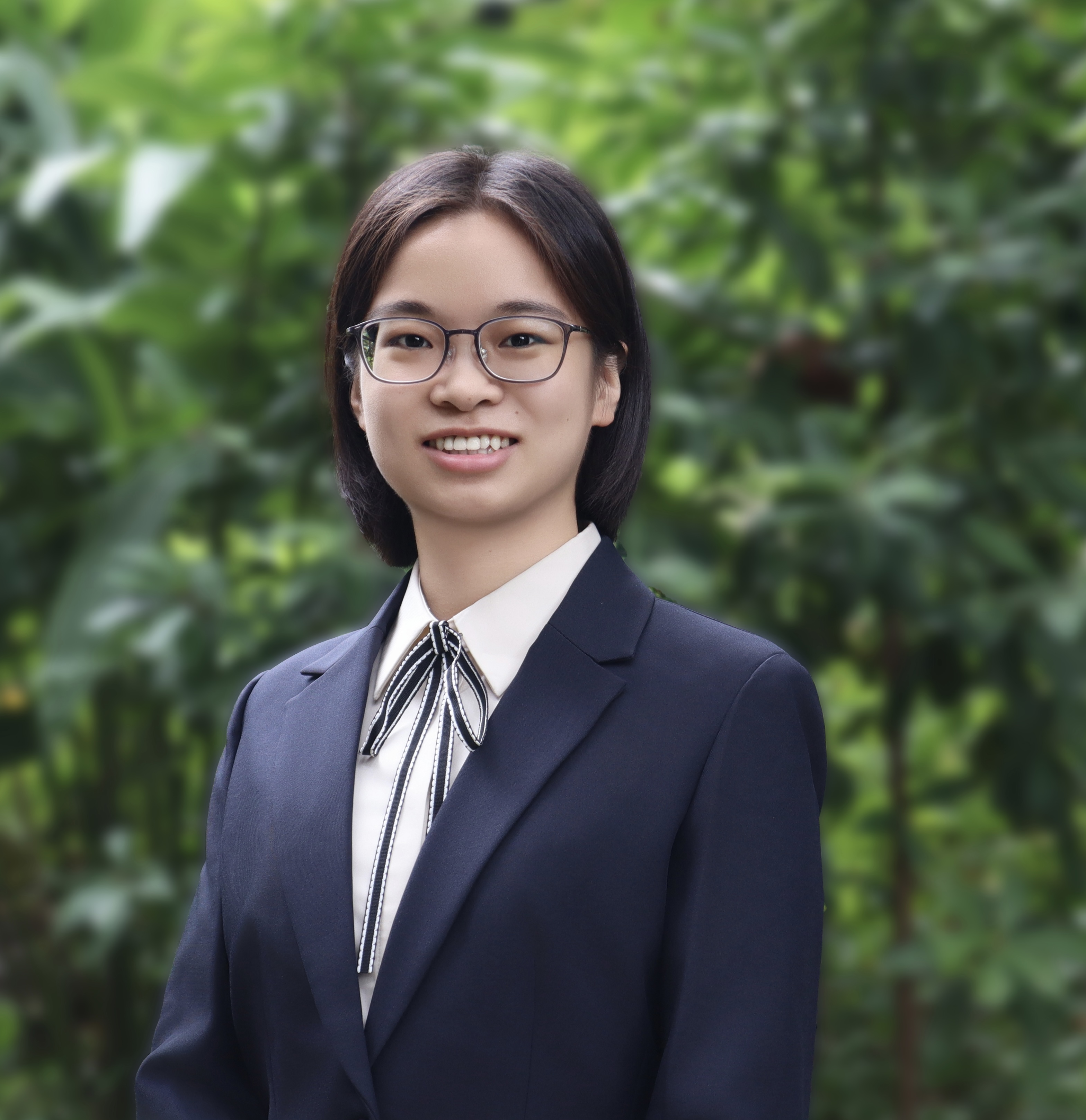
WANG KEXIN
Ph.D Candidate in Economics
I am a job market candidate and I will be available for interviews.
REFERENCES
Professor Jiangtao Li
Email: tli@smu.edu.sg
Tel: +65 68261351
Professor Wei He
Email: hewei@cuhk.edu.hk
Tel: (852) 3943-8196
Professor Jingyi Xue
Email: jyxue@smu.edu.sg
Tel: +65 68085466
Professor Tilman Börgers
Email: tborgers@umich.edu
Tel: +-1-734-764 8022
WORKING PAPERS
"Robust Contracting under Distributional Uncertainty", with Jiangtao Li (Job Market Paper)
We study the design of contracts when the principal has limited statistical information about the output distributions induced by the agent’s actions. In the baseline model, we consider a principal who only knows the mean of the output distribution for each action. The mean restrictions allow for large set of profiles of output distributions, including some extreme output distributions that can be used to establish the robust optimality of monotone affine contracts. Motivated by this, we study the set of distributions that can be used to establish the robust optimality of the monotone affine contracts. This facilitates the understanding for the use of monotone affine contracts in settings with more restrictions on the output distributions. Our main result shows that the optimality of monotone affine contracts persists even if the principal has access to other information about the output distributions, such as the information that the output distribution induced by each action has full support.
RESEARCH PAPERS
"A Robust Optimization Approach to Mechanism Design", with Jiangtao Li
We study the design of mechanisms when the mechanism designer faces local uncertainty about agents’ beliefs. Specifically, we consider a designer who does not know the exact beliefs of the agents but is confident that her estimate is within ε of the beliefs held by the agents (where ε reflects the degree of local uncertainty). Adopting the robust optimization approach, we design mechanisms that incentivize agents to truthfully report their payoff-relevant information regardless of their actual beliefs. For any fixed ε, we identify necessary and sufficient conditions under which requiring this sense of robustness is without loss of revenue for the designer. By analyzing the limiting case in which ε approaches 0, we provide two rationales for the widely studied Bayesian mechanism design framework.
“Interim Regret Minimization”, with Wei He, Jiangtao Li
We consider a robust version of monopoly pricing when the seller only knows the bound on valuations and the mean of the distribution of the buyer’s value. The seller seeks to minimize interim regret, the forgone expected revenue due to not knowing the distribution of the buyer’s value. The optimal pricing policy randomizes over a range of prices; the support of the pricing policy is bounded away from zero.
“Undominated Mechanisms”, with Tilman Börgers, Jiangtao Li
We study the design of mechanisms when the designer faces multiple plausible scenarios and is uncertain about the true scenario. A mechanism is dominated by another if the latter performs at least as well in all plausible scenarios and strictly better in at least one. A mechanism is undominated if no other feasible mechanism dominates it. We show how analyzing undominated mechanisms could be useful and illustrate the tractability of characterizing such mechanisms. This approach provides an alternative criterion for mechanism design under uncertainty, complementing existing methods.
“Optimal Multiperiod Allocation with Costly Verification”
We study the optimal design of mechanisms in which the principal is to allocation goods to an agent over two periods. In each period, the principal can verify the agent’s private information at a cost, but monetary transfers are not allowed. In particular, we consider that the agent’s private value for a good is either high or low in each period. And the values are independent and identically distributed over two periods. In our main results, we first show that it is always suboptimal to check the agent’s information for sure in the first period when he reports the high value. This contrasts with the optimality for the static allocation problem that has been studied in Ben-Porath, Dekel, and Lipman (2014), in which verifying the high value with probability one maximizes the principal’s expected payoff when certain conditions are satisfied. Second, we explicitly solve out the optimal mechanisms, which strongly depend on the choice of model parameters, including the valuations, the verification cost as well as the value distributions. We also discuss the optimal mechanisms when the agent’s values exhibit two extreme correlation structures, which are maximal positive correlation or maximal negative correlation.