|
TOPIC:
|
A RANDOMIZED MISSING DATA APPROACH TO ROBUST FILTERING WITH APPLICATIONS TO ECONOMICS AND FINANCE
|
ABSTRACT
We put forward a simple new approach to robust filtering of state-space models, motivated by the idea that the inclusion of only a small fraction of available highly precise measurements can still extract most of the attainable efficiency gains for filtering latent states, estimating model parameters, and producing out-of-sample forecasts. The new class of particle filters we develop aims to achieve a degree of robustness to outliers and model misspecification by purposely randomizing the subset of utilized highly precise but possibly misspecified or outlier contaminated data measurements, while treating the rest as if missing. The arising robustness-efficiency trade-off is controlled by varying the fraction of randomly utilized measurements or the incurred relative efficiency loss from such randomized utilization of the available measurements. As an empirical illustration, we consider popular state space models for inflation and equity returns with stochastic volatility and document favorable performance of our robust particle filter and density forecasts on both simulated and real data. More generally, our randomization approach makes it easy to robustly incorporate highly informative but possibly contaminated modern “big data” streams for improved state-space filtering and forecasting.
Keywords: State space models, particle filtering, robustness, outliers, misspecification, stochastic volatility, realized volatility, return density forecasting, inflation forecasting.
Click here to view the paper.
Click here to view the CV.
|
|
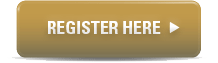
|
PRESENTER
Dobrislav Dobrev
Federal Reserve Board
|
RESEARCH FIELDS
Financial Econometrics in Data-Rich Environments
High Frequency Trading
|
DATE:
4 February 2020 (Tuesday)
|
TIME:
4pm - 5.30pm
|
VENUE:
Meeting Room 5.1, Level 5
School of Economics
Singapore Management University
90 Stamford Road
Singapore 178903
|
|
|