|
TOPIC:
|
LIMIT THEORY FOR MULTIVARIATE LINEAR DIFFUSION ESTIMATION
|
ABSTRACT
This paper provides a new representation of the maximum likelihood (ML) estimator of the "mean reversion matrix" in the multivariate Ornstein-Uhlenbeck process. The new representation enjoys three advantages over the traditional matrix logarithmic representation. First, while the traditional matrix logarithmic representation involves an infinite polynomial series, the new representation involves only finite polynomial series whose degree is the same as the dimension of the multivariate process. This formulation avoids truncation of an infinite series and facilitates the use of the delta method and the calculation of the covariance matrix in the limit distribution. Second, the new representation has a wider domain of convergence than the traditional series. Third, the new representation readily accommodates prior knowledge about the model (e.g., the cointegrating rank) to simplify the estimating procedure, whereas it is hard to do so with the traditional series approach. The limit theory of the ML estimator based on the new representation is established for a wide range of cases, including stationary, pure unit root, or partially nonstationary processes. Special attention is given to provide an explicit expression of the asymptotic covariance matrix for low dimensional models. An empirical application is conducted on an affine term structure model, illustrating the advantages of the new representation. Although the theory is derived for linear diffusions, the method proposed in the paper is applicable to nonlinear diffusion models.
JEL Classification: C13, C32, G12
Keywords: Multivariate diffusions; Exact maximum likelihood estimator; Principal logarithm; Limit theory; Stationarity and non-stationarity
Click here to view paper.
|
|
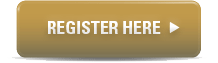
|
PRESENTER
Wang Xiaohu
The Chinese University of Hong Kong
|
RESEARCH FIELDS
Econometric Theory
Financial Econometrics
Time Series Analysis
|
DATE:
27 July 2016 (Wednesday)
|
TIME:
4pm - 5.30pm
|
VENUE:
Meeting Room 5.1, Level 5
School of Economics
Singapore Management University
90 Stamford Road
Singapore 178903
|
|
|