|
TOPIC:
|
LIKELIHOOD BASED INFERENCE FOR DYNAMIC PANEL DATA MODELS
|
ABSTRACT
This paper considers maximum likelihood (ML) based inferences for dynamic panel data models. We focus on the analysis of the panel data with a large number (N) of cross-sectional units and a small number (T) of repeated time-series observations for each cross-sectional unit. We examine several different ML estimators and their asymptotic and finite-sample properties. Our major finding is that when data follow unit-root processes without or with drifts, the ML estimators have singular information matrices. This is a case of Sargan (1983) in which the first order condition for identification fails, but parameters are identified. The ML estimators are consistent, but they have nonstandard asymptotic distributions and their convergence rates are lower than N1/2. In addition, the sizes of usual Wald statistics based on the estimators are distorted even asymptotically, and they reject the unit-root hypothesis too often. However, following Rotnitzky, Cox, Bottai and Robins (2000), we show that likelihood ratio (LR) tests for unit root follow mixtures of chi-square distributions. Our Monte Carlo experiments show that the LR tests with the p-values from the mixed distributions are much better sized than the Wald tests, although they tend to slightly over-reject the unit root hypothesis in small samples. It is also shown that the LR tests for unit roots have good finite-sample power properties.
Keywords: Dynamic Panel Data, Maximum Likelihood, Singular Information Matrix.
JEL Codes: C23, C40.
Click here to view the paper.
Click here to view the CV.
|
|
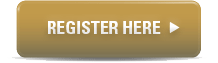
|
PRESENTER
Seung Anh
Arizona State University
|
RESEARCH FIELDS
Econometrics
Econometric Modeling
|
DATE:
22 May 2019 (Wednesday)
|
TIME:
4pm - 5.30pm
|
VENUE:
Meeting Room 5.1, Level 5
School of Economics
Singapore Management University
90 Stamford Road
Singapore 178903
|
|
|