|
TITLE:
|
Three Essays on Nonstationary Time-series Analysis and Network Dynamics
|
ABSTRACT
This dissertation consists of three essays which contribute new theoretical results to nonstationary time-series analysis and network dynamics. In Chapter 2, we develop an asymptotic theory for distinguishing explosive models with the unit root model using information criteria. Both OLS estimator and indirect inference estimator are studied in all three types of explosive models. First, we find that information criteria may consistently choose the unit root model under cases where the autoregressive coefficient depends on the sample size. Second, we prove that the validity of using information criteria for explosive model selection against unit root depends crucially on both the speed of autoregressive coefficient converging to unity and the speed of information criteria specific penalty converging to zero as sample size goes to infinity. In Chapter 3, we study a continuous time dynamic system with a random persistence parameter. The model distinguishes various forms of unstable and explosive behaviour according to specific regions of the parameter space that open up the potential for testing these forms of extreme behaviour. A two-stage approach that employs realized volatility is proposed for the continuous system estimation, asymptotic theory is developed, and test statistics to identify the different forms of extreme sample path behaviour are proposed. An empirical application based on daily real S&P 500 index data over 1964-2015 reveals strong evidence against parameter constancy after early 1980, which strengthens after July 1997, leading to a long duration of what the model characterizes as extreme behaviour in real stock prices. In Chapter 4, we develop a dynamic covariate-assisted spectral clustering method to uniformly estimate the latent group membership of cryptocurrencies consistently. We show that return inter-predictability and crypto characteristics, including hashing algorithms and proof types, jointly determine the crypto market segmentation. Based on this classification result, it is natural to employ eigenvector centrality to identify a cryptocurrency’s idiosyncratic risk. An asset pricing analysis finds that a cross-sectional portfolio with a higher centrality earns a higher risk premium. Further tests confirm that centrality serves as a risk factor well and delivers valuable information content on cryptocurrency markets.
|
|
PRESENTER
TAO Yubo
PhD Candidate
School of Economics
Singapore Management University
|
|
DISSERTATION COMMITTEE:
Chair:
Professor Peter C. B. PHILLIPS
Distinguished Term Professor of Economics
Lee Kong Chian Fellow
Co-Chair:
Professor YU Jun
Lee Kong Chian Professor of Economics and Finance
Committee Members:
Professor Yichong ZHANGT
Assistant Professor of Economics
External Member:
Professor Anastasios MAGDALINOS
Professor of Econometrics
|
RESEARCH FIELDS
Financial Econometrics, Econometric Theory
|
DATE:
3 May 2019 (Friday)
|
TIME:
10.30am
|
VENUE:
Interactive Learning Space, Level 5
School of Economics
Singapore Management University
90 Stamford Road
Singapore 178903
|
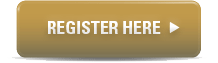
|
|
|