showSidebars ==
showTitleBreadcrumbs == 1
node.field_disable_title_breadcrumbs.value ==
SMU SOE Seminar Series (March 22, 2024): Scalable and Hyper-parameter-free Non-parametric Covariate Shift Adaptation with Conditional Sampling
|
TOPIC: | SCALABLE AND HYPER-PARAMETER-FREE NON-PARAMETRIC COVARIATE SHIFT ADAPTATION WITH CONDITIONAL SAMPLING |
ABSTRACTMany existing covariate shift adaptation methods estimate sample weights to be used in the risk estimation in order to mitigate the gap between the source and the target distribution. However, non-parametrically estimating the optimal weights typically involves computationally expensive hyper-parameter tuning that is crucial to the final performance. In this paper, we propose a new non-parametric approach to covariate shift adaptation which avoids estimating weights and has no hyper-parameter to be tuned. Our basic idea is to label unlabeled target data according to the k-nearest neighbors in the source dataset. Our analysis indicates that setting k = 1 is an optimal choice. Thanks to this property, there is no need to tune any hyper-parameters, unlike other non-parametric methods. Moreover, our method achieves a running time quasi-linear in the sample size with a theoretical guarantee, for the first time in the literature to the best of our knowledge. Our results include sharp rates of convergence for estimating the joint probability distribution of the target data. In particular, the variance of our estimators has the same rate of convergence as for standard parametric estimation despite their non-parametric nature. Our numerical experiments show that proposed method brings drastic reduction in the running time with accuracy comparable to that of the state-of-the-art methods. Click here to view the CV. Click here to view the paper. | | 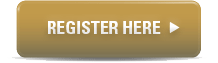 | PRESENTER | RESEARCH FIELDSStatistics for Dependent Data Time Series Analysis Markov Chains | DATE:22 March 2024 (Friday) | TIME:4pm - 5.30pm | VENUE:Meeting Room 5.1, Level 5 School of Economics Singapore Management University 90 Stamford Road Singapore 178903 |
|
|
|