|
TOPIC:
|
LARGE SYSTEM OF SEEMINGLY UNRELATED REGRESSIONS: A PENALIZED QUASI-MAXIMUM LIKELIHOOD ESTIMATION PERSPECTIVE
|
ABSTRACT
In this paper, we propose using a shrinkage estimator, penalized quasi-maximum likelihood estimator (PQMLE), to estimate a large system of equations in seemingly unrelated regressions model where the number of equations is large relative to sample size. We develop the asymptotic properties of the penalized quasi-maximum likelihood estimator for both error covariance matrix and model coefficients. In particular, we derive the asymptotic distribution of the coefficient estimator and the convergence rate of the estimated covariance matrix in terms of Frobenius norm. The model selection consistency property of the covariance matrix estimator is also established. Simulation results show that when the number of equations is large relative to sample size and the error covariance matrix is sparse, the penalized likelihood estimator performs much better than conventional estimators. As an illustration, we apply the PQMLE to the study of state level public capital returns in the United States.
Keywords: Seemingly unrelated regression, Large covariance matrix, Shrinkage estimation
JEL Classification: C13, C33, C55
Click here to view his CV.
|
|
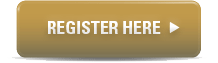
|
PRESENTER
Qingliang Fan (Michael)
Xiamen University
|
RESEARCH FIELDS
Labor Economics
Environmental Economics
Financial Economics
|
DATE:
17 Mar 2017 (Friday)
|
TIME:
4pm - 5.30pm
|
VENUE:
Meeting Room 5.1, Level 5
School of Economics
Singapore Management University
90 Stamford Road
Singapore 178903
|
|
|