showSidebars ==
showTitleBreadcrumbs == 1
node.field_disable_title_breadcrumbs.value ==
SMU SOE Seminar (Mar 15, 2019): Normal Approximation in Large Network Models
|
TOPIC:
|
NORMAL APPROXIMATION IN LARGE NETWORK MODELS
|
ABSTRACT
We prove central limit theorems under large-network asymptotics for empirical models of network formation and network processes with homophilous agents. These results enable inference in a large class of network models in the typical setting where the sample consists of a small set of large networks. We first establish a central limit theorem under high-level ``stabilization'' conditions that provide a general and useful formulation of weak dependence, particularly in models with strategic interactions. The result delivers a $\sqrt{n}$ rate of convergence and a closed-form expression for the asymptotic variance. Using techniques in branching process theory, we derive primitive conditions for stabilization in the following applications: static and dynamic models of strategic network formation, network regressions, and treatment effects with network spillovers. Finally, we discuss practical methods for inference, including a HAC-type variance estimator.
Click here to view the CV.
|
|
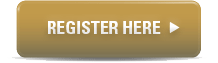
|
PRESENTER
Michael Leung
University of Southern California
|
RESEARCH FIELDS
Econometrics
Networks
|
DATE:
15 March 2019 (Friday)
|
TIME:
4pm - 5.30pm
|
VENUE:
Meeting Room 5.1, Level 5
School of Economics
Singapore Management University
90 Stamford Road
Singapore 178903
|
|
|
|