showSidebars ==
showTitleBreadcrumbs == 1
node.field_disable_title_breadcrumbs.value ==
SMU SOE Seminar (February 23, 2024, 4pm-5.30pm): Production Function Estimation Using Subjective Expectations Data
|
TOPIC: | PRODUCTION FUNCTION ESTIMATION USING SUBJECTIVE EXPECTATIONS DATA |
ABSTRACTStandard methods for estimating production functions in the Olley and Pakes (1996) tradition require assumptions on input choices. We introduce a new method that exploits expectations data, which is increasingly available, and allows us to relax these input demand assumptions to obtain consistent production function parameter estimates. In contrast to dynamic panel data methods, our proposed estimator can be implemented on very short panels (including a single cross-section), and Monte Carlo simulations show it outperforms alternative estimators when firms' material and/or investment choices are subject to optimization error. Implementing a range of production function estimators on UK data, we find our proposed estimator obtains larger estimates of firm-level productivity dispersion. Consistent with evidence from the US, we find that productivity dispersion increased between 2000 and 2020 while firm employment became less responsive to productivity shocks. These results are robust to the choice of production function estimator and evidence that misallocation has increased, contributing to the slowdown in productivity growth in recent years. Click here to view the CV. | | 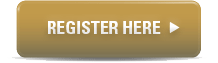 | PRESENTERAureo de Paula University College London | RESEARCH FIELDSMicroeconometrics Empirical Microeconomics | DATE:23 February 2024 (Friday) | TIME:4pm - 5.30pm | VENUE:Meeting Room 5.1, Level 5 School of Economics Singapore Management University 90 Stamford Road Singapore 178903 |
|
|
|