|
TOPIC:
|
LARGE DYNAMIC COVARIANCE MATRICES
|
ABSTRACT
Second moments of asset returns are important for risk management and portfolio selection. The problem of estimating second moments can be approached from two angles: time series and the cross-section. In time series, the key is to account for conditional heteroskedasticity; a favored model is Dynamic Conditional Correlation (DCC), derived from the ARCH/GARCH family started by Engle (1982). In the cross-section, the key is to correct in-sample biases of sample covariance matrix eigenvalues; a favored model is nonlinear shrinkage, derived from Random Matrix Theory (RMT). The present paper aims to marry these two strands of literature in order to deliver improved estimation of large dynamic covariance matrices.
Keywords: Composite likelihood, dynamic conditional correlations, GARCH,
Markowitz portfolio selection, nonlinear shrinkage.
JEL Classification: C13, C58, G11
Click here to view the paper.
Click here to view his CV.
|
|
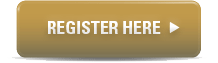
|
PRESENTER
Michael Wolf
University of Zurich
|
RESEARCH FIELDS
Nonparametric Inference Methods,
Multiple Testing Procedures,
Financial Econometrics,
Large-dimensional Covariance Matrices
|
DATE:
1 Feb 2017 (Wednesday)
|
TIME:
4pm - 5.30pm
|
VENUE:
Meeting Room 5.1, Level 5
School of Economics
Singapore Management University
90 Stamford Road
Singapore 178903
|
|
|