showSidebars ==
showTitleBreadcrumbs == 1
node.field_disable_title_breadcrumbs.value ==
SMU SOE Online Seminar (Oct 4, 2021, 9.00am-10.30am):Inference in Regression Discontinuity Designs under Monotonicity
|
TOPIC:
|
INFERENCE IN REGRESSION DISCONTINUITY DESIGNS UNDER MONOTONICITY
|
ABSTRACT
We provide an inference procedure for the sharp regression discontinuity design (RDD) under monotonicity, with possibly multiple running variables. Specifically, we consider the case where the true regression function is monotone with respect to (all or some of) the running variables and assumed to lie in a Lipschitz smoothness class. Such a monotonicity condition is natural in many empirical contexts, and the Lipschitz constant has an intuitive interpretation. We propose a minimax two-sided confidence interval (CI) and an adaptive one-sided CI. For the two-sided CI, the researcher is required to choose a Lipschitz constant where she believes the true regression function to lie in. This is the only tuning parameter, and the resulting CI has uniform coverage and obtains the minimax optimal length. The one-sided CI can be constructed to maintain coverage over all monotone functions, providing maximum credibility in terms of the choice of the Lipschitz constant. Moreover, the monotonicity makes it possible for the (excess) length of the CI to adapt to the true Lipschitz constant of the unknown regression function. Overall, the proposed procedures make it easy to see under what conditions on the underlying regression function the given estimates are significant, which can add more transparency to research using RDD methods.
Click here to view the CV.
|
|
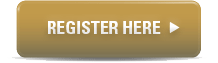
This seminar will be held virtually via Zoom. A confirmation email with the Zoom details will be sent to the registered email by 1 October 2021.
|
PRESENTER
Soonwoo Kwon
Brown University
|
RESEARCH FIELDS
Econometric Theory
Applied Econometrics
|
DATE:
4 October 2021 (Monday)
|
TIME:
9.00am - 10.30am
|
|
|
|