showSidebars ==
showTitleBreadcrumbs == 1
node.field_disable_title_breadcrumbs.value ==
Nonstationary Cross-Validation
|
TOPIC:
|
nonstationary cross-validation
|
ABSTRACT
Cross-validation is the most common data-driven procedure for choosing the bandwidth sequence in nonparametric regression. For the case of i.i.d or strong mixing data, it is well-known that the bandwidth chosen by cross-validation is optimal with respect to the mean integrated squared error. However, the properties of cross-validated bandwidths in the context of nonstationary regressions have not yet been established. This is the subject of the current paper. For the case of β-recurrent (stationary or nonstationary) Markov chains, we show that the bandwidth chosen via cross-validation is optimal with respect to the average squared error. The accuracy of estimators based on cross-validated bandwidths is analyzed via a Monte Carlo study. The practical usefulness of cross-validated bandwidths in a highly-persistent, possibly nonstationary environment is illustrated by virtue of an application to nonlinear predictive regressions.
Click here to view paper.
|
|
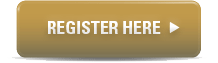
|
Presenter
|
Research Fields
Econometric Theory, Financial Econometrics, Time Series: Predictive Evaluation
|
Date:
18 Sept 2015 (Friday)
|
Time:
4pm - 5.30pm
|
Venue:
Meeting Room 5.1, Level 5
School of Economics
Singapore Management University
90 Stamford Road
Singapore 178903
|
|
|
|
|