Machine learning in forecast combinations
Synopsis
Reliable forecasting based on observational evidence is useful in individual and firm decision-making, as well as governmental planning, regulation, and many other activities that rely on future economic projections. When structural instabilities and nonstationarities are present in data, a common approach in the econometrics literature is to combine forecasts from different econometric models. This technique is known as forecast combinations. In economics, structural instabilities and nonstationarities are widely expected as the economic environment changes constantly.
In recent years, machine learning (ML) methods have found successful applications in predicting economic activities, especially when the underlying relationship linking response and explanatory variables is complicated. However, ML methods typically assume stability in the underlying relationship. Hence, existing ML methods may not be suitable for making economic forecasting when data involve structural instabilities and nonstationarities.
In this lecture, we propose a novel framework that first allows machine learning methods to simultaneously consider multiple model specification, and then averages the outputs by the weights that are either pre-specified or estimated. In empirical applications to forecast key macroeconomic variables (such as GDP growth, the inflation rate, and the unemployment rate) and financial variables (such as the interest rate), we find that combining machine learning methods can produce more accurate forecasts than individual machine learning techniques and traditional econometric methods.
About the Speaker
Professor Jun Yu
Lee Kong Chian Professor of Economics and Finance
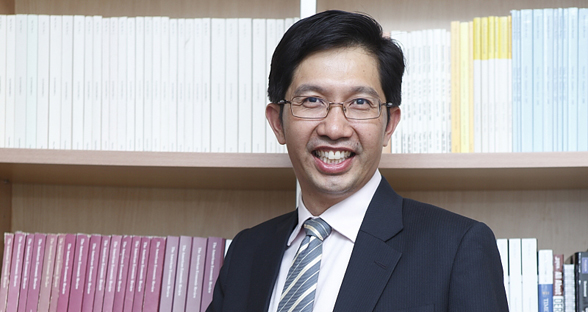
Professor Jun Yu joined SMU in January 2004. He is currently Lee Kong Chian Professor of Economics and Finance at SMU. Professor Yu was previously the Director of SMU’s Sim Kee Boon Institute for Financial Economics and Co-Director of its Centre for Financial Econometrics.
Professor Yu has published more than 70 papers in academic journals and handbooks. Many of these publications are in leading journals in economics, finance, and econometrics. His articles with Professors Peter Phillips, Yanru Wu and Shuping Shi, for detecting the presence of asset price bubbles and estimating their origination and termination dates, have initiated a new area of research on the econometric analysis of bubbles in financial assets and real estate. Large numbers of researchers have been attracted to work in this area using the methods developed in these articles. Many central banks have used these techniques for early warning signals. Several computer software packages have been written to implement these methods. In 2020, his co-authored book, titled “Financial Econometric Modeling”, was published by Oxford University Press.
Professor Yu has been awarded external research grants from the MOE Academic Research Fund Tier-3 grant and two MOE Tier-2 grants. He was the lead Principal-Investigator of the Tier-3 programme on the economics of ageing.
Professor Yu holds a PhD in Economics from University of Western Ontario, and a BSc in Mathematics and BA in Economics from Wuhan University. He is a Fellow of the Journal of Econometrics and also a Founding Fellow of the Society of Financial Econometrics. He serves as Associate Editor of the Journal of Econometrics, Econometric Theory and Journal of Financial Econometrics.
Click
here to view the CV.
Event Details
Date: 8 December 2020, Tuesday
Time: 10.00 am - 11.00 am (Singapore Time)
Registration
This lecture will be held via Zoom. A confirmation email with the Zoom details will be sent to the registered email by 4 December 2020.
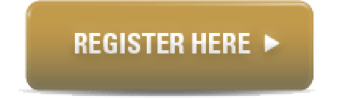