showSidebars ==
showTitleBreadcrumbs == 1
node.field_disable_title_breadcrumbs.value ==
Gradient Based Smoothing Parameter Selection for Nonparametric Regression Estimation
|
TOPIC:
|
Gradient Based Smoothing Parameter Selection for Nonparametric Regression Estimation
|
ABSTRACT
Uncovering gradients is of crucial importance across a broad range of economic environments. Here we consider data-driven bandwidth selection based on the gradient of an unknown regression function. The procedure developed here is automatic and does not require initial estimation of unknown functions with pilot bandwidths, and we prove that it delivers bandwidths which have the optimal rate of convergence for the gradient. An important advantage of our proposed method is that it overcomes the tendency of traditional data-driven approaches to engage in under smoothing. Both simulated and empirical examples showcase the finite sample attraction of this new mechanism.
Please click here to download paper.
|
|
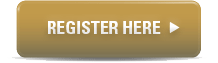
|
Presenter
Daniel Henderson
The University of Alabama
|
Research Fields
Nonparametric Econometrics, Panel Data Econometrics, Applied Microeconometrics, Economic Growth and Development, Economics of Education
|
Date:
13 September 2013 (Friday)
|
Time:
12pm - 1.30pm
|
Venue:
Seminar Room 5.1, Level 5
School of Economics
Singapore Management University
90 Stamford Road
Singapore 178903
|
|
|
|
|