showSidebars ==
showTitleBreadcrumbs == 1
node.field_disable_title_breadcrumbs.value ==
Essays on High-Frequency Financial Data Analysis
|
Title:
|
Essays on High Frequency Financial Data Analysis
|
ABSTRACT
This dissertation consists of three essays on high-frequency financial data analysis. I consider intraday periodicity adjustment and its effect on intraday volatility estimation, the Business Time Sampling (BTS) scheme and the estimation of market microstructure noise using NYSE tick-by-tick transaction data. Chapter 2 studies two methods of adjusting for intraday periodicity of high-frequency financial data: the well-known Duration Adjustment (DA) method and the recently proposed Time Transformation (TT) method (Wu (2012)). I examine the effects of these adjustments on the estimation of intraday volatility using the Autoregressive Conditional Duration-Integrated Conditional Variance (ACD-ICV) method of Tse and Yang (2012). I find that daily volatility estimates are not sensitive to intraday periodicity adjustment. However, intraday volatility is found to have a weaker U-shaped volatility smile and a biased trough if intraday periodicity adjustment is not applied. In addition, adjustment taking account of trades with zero duration (multiple trades at the same time stamp) results in deeper intraday volatility smile. Chapter 3 proposes a new method to implement the Business Time Sampling (BTS) scheme for high-frequency financial data using a time-transformation function. The sampled BTS returns have approximately equal volatility given a target average sampling frequency. My Monte Carlo results show that the Tripower Realized Volatility (TRV) estimates of daily volatility using the BTS returns produce smaller root mean-squared error than estimates using returns based on the Calendar Time Sampling (CTS) and Tick Time Sampling (TTS) schemes, with and without subsampling. Based on the BTS methodology I propose a modified ACD-ICV estimate of intraday volatility and find that this new method has superior performance over the Realized Kernel estimate and the ACD-ICV estimate based on sampling by price events. Chapter 4 proposes new methods to estimate the noise variance of high-frequency stock returns using differences of subsampling realized variance estimates at two or multiple time scales. Noise-variance estimates are compared and my proposed estimates perform the best in reporting lower mean error (ME) and root mean-squared error (RMSE). My study shows significant estimation error for noise-variance estimates when transactions are selected at too high or too low frequencies. For a typical NYSE stock, the NSR is around 0.005% in the period from 2010 to 2013. I also find negative cross correlation between noise and latent asset returns in my empirical study.
|
|
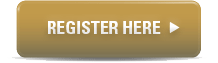
|
Presenter
Dong Yingjie Singapore Management University
|
Research Fields
Financial Econometrics, High-Frequency Data Analysis
|
Date:
20th April 2015
|
Time:
9.00am - 11.00am
|
Venue:
CREA Meeting Room at Level 6
School of Economics
Singapore Management University
90 Stamford Road
Singapore 178903
|
|
|
|
|