|
TOPIC:
|
MISSPECIFIED BAYESIAN LEARNING BY STRATEGIC PLAYERS: FIRST-ORDER MISSPECIFICATION AND HIGHER-ORDER MISSPECIFICATION
|
ABSTRACT
We consider strategic players who may have a misspecified view about the world, and investigate their long-run behavior when they learn an unknown state from public signals over time. Our framework is flexible and allows for higher-order misspecification, in that a player may have a bias about the physical environment, a bias about the opponent's bias about the physical environment, and so on. We provide a condition under which players' beliefs and actions converge to a steady state, and then characterize how one's misspecification influences the long-run (steady-state) outcome.We apply these results to various economic examples such as Cournot competition, team production, and discrimination to study when one's misspecifcation improves her own payoff and how it influences the opponent's behavior. We also find that higher-order misspecification can have a significant impact on the equilibrium outcome, e.g., one's bias about the physical environment can have opposite effects on their payoffs and actions, depending on whether the opponent is aware of this bias or not.
Click here to view the CV.
|
|
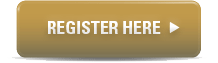
This seminar will be held virtually via Zoom. A confirmation email with the Zoom details will be sent to the registered email by 8 September 2021.
|
PRESENTER
Yuichi Yamamoto
Hitotsubashi University
|
RESEARCH FIELDS
Microeconomic Theory
|
DATE:
9 September 2021 (Thursday)
|
TIME:
4.00pm - 5.30pm
|
|
|