|
TOPIC:
|
BAYESIAN ESTIMATION AND COMPARISON OF CONDITIONAL MOMENT MODELS
|
ABSTRACT
We provide a Bayesian analysis of models in which the unknown distribution of the outcomes is specified up to a set of conditional moment restrictions. This analysis is based on the nonparametric exponentially tilted empirical likelihood (ETEL) function, which is constructed to satisfy a sequence of unconditional moments, obtained from the conditional moments by an increasing (in sample size) vector of approximating functions (such as tensor splines based on the splines of each conditioning variable). The posterior distribution is shown to satisfy the Bernstein-von Mises theorem, subject to a growth rate condition on the number of approximating functions, even under misspecification of the conditional moments. A large-sample theory for comparing different conditional moment models is also developed. The central result is that the marginal likelihood criterion selects the model that is less misspecified, that is, the model that is closer to the unknown true distribution in terms of the Kullback-Leibler divergence. Several examples are provided to illustrate the framework and results.
Keywords: Bayesian inference, bernstein-von mises theorem, conditional moment restrictions, ex-ponentially tilted empirical likelihood, marginal likelihood, misspecification, posterior consistency.
JEL Codes: C11, C14, C13, C52.
Click here to view the CV.
|
|
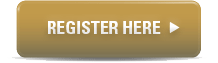
This seminar will be held via Zoom. A confirmation email with the Zoom details will be sent to the registered email by 15 October 2020.
|
PRESENTER
Anna Simoni
Ecole Polytechnique
|
RESEARCH FIELDS
Econometrics
High Dimensional Econometrics
Bayesian Statistics
Google Search Data
|
DATE:
16 October 2020 (Friday)
|
TIME:
4.00pm - 5.30pm
|
|
|