|
TOPIC:
|
MACHINE LEARNING'S DROPOUT TRAINING IS DISTRIBUTIONALLY ROBUST OPTIMAL
|
ABSTRACT
Dropout training is an increasingly popular estimation method in machine learning that minimizes some given loss function (e.g., the negative expected log-likelihood), but averaged over nested submodels chosen at random. This paper shows that dropout training in Generalized Linear Models is the minimax solution of a two-player, zero-sum game where an adversarial nature corrupts a statistician’s covariates using a multiplicative nonparametric errors-in-variables model. In this game—known as a Distributionally Robust Optimization problem—nature’s least favorable distribution is dropout noise, where nature independently deletes entries of the covariate vector with some fixed probability δ. Our decision-theoretic analysis shows that dropout training—the statistician’s minimax strategy in the game—indeed provides out-of-sample expected loss guarantees for distributions that arise from multiplicative perturbations of in-sample data. This paper also provides a novel, parallelizable, Unbiased Multi-Level Monte Carlo algorithm to speed-up the implementation of dropout training. Our algorithm has a much smaller computational cost compared to the naive implementation of dropout, provided the number of data points is much smaller than the dimension of the covariate vector.
Keywords: Generalized linear models, distributionally robust optimization, machine learning, minimax theorem, multi-level monte carlo.
Click here to view the paper.
Click here to view the CV.
|
|
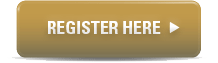
This seminar will be held via Zoom. A confirmation email with the Zoom details will be sent to the registered email by 1 October 2020.
|
PRESENTER
Jose Luis Montiel Olea
Columbia University
|
RESEARCH FIELDS
Econometrics
Machine Learning
|
DATE:
2 October 2020 (Friday)
|
TIME:
9.00am - 10.30am
|
|
|