|
TOPIC:
|
THE WILD BOOTSTRAP WITH A "SMALL" NUMBER OF "LARGE" CLUSTERS
|
ABSTRACT
This paper studies the properties of the wild bootstrap-based test proposed in Cameron et al. (2008) in settings with clustered data. Cameron et al. (2008) provide simulations that suggest this test works well even in settings with as few as five clusters, but existing theoretical analyses of its properties all rely on an asymptotic framework in which the number of clusters is “large.” In contrast to these analyses, we employ an asymptotic framework in which the number of clusters is “small,” but the number of observations per cluster is “large.” In this framework, we provide conditions under which the limiting rejection probability of an un-Studentized version of the test does not exceed the nominal level. Importantly, these conditions require, among other things, certain homogeneity restrictions on the distribution of covariates. We further establish that the limiting rejection probability of a Studentized version of the test does not exceed the nominal level by more than an amount that decreases exponentially with the number of clusters. We study the relevance of our theoretical results for finite samples via a simulation study.
Keywords: Wild Bootstrap, Clustered Data, Randomization Tests.
Click here to view the paper.
Click here to view the CV.
|
|
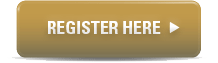
|
PRESENTER
Andres Santos
University of California, Los Angeles
|
RESEARCH FIELDS
Econometrics
|
DATE:
8 May 2019 (Wednesday)
|
TIME:
4pm - 5.30pm
|
VENUE:
Meeting Room 5.1, Level 5
School of Economics
Singapore Management University
90 Stamford Road
Singapore 178903
|
|
|