|
TOPIC:
|
ROBUST ASYMPTOTIC INFERENCE ABOUT CONDITIONAL TAIL PROPERTIES: A PANEL DATA APPROACH
|
ABSTRACT
I consider inference about conditional tail properties such as conditional tail index and conditional extremal quantile. Most existing suggestions with extremal quantile regression as the leading approach rely on the key assumption that the tail shape of the underlying conditional distribution remains unchanged given different conditional values, implying that the conditional extremal quantile can be approximated by a location-scale model. However, this assumption holds only in limited cases such as joint normal distribution, but might be violated in many other empirically relevant cases such as joint student’s t distribution. To construct robust inference with imposing only mild regularity conditions, I develop asymptotically valid confidence intervals for conditional tail properties based on panel data and extreme value theory. These intervals allow for unobserved heterogeneity and dynamic panel, and have excellent small sample coverage and length properties. To illustrate their empirical use, I study (i) tail risk of the U.S. stock return given stock size and (ii) the extremely low quantile of infant birthweight given mother’s net weight gain during pregnancy.
Keywords: Panel data, Conditional tail, Extreme value theory, Nonparametric confidence interval
Click here to view the CV.
|
|
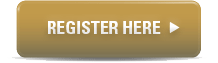
|
PRESENTER
Yulong Wang
Syracuse University
|
RESEARCH FIELDS
Econometrics
Applied econometrics
Risk management
Finance
|
DATE:
14 March 2019 (Thursday)
|
TIME:
4pm - 5.30pm
|
VENUE:
Meeting Room 5.1, Level 5
School of Economics
Singapore Management University
90 Stamford Road
Singapore 178903
|
|
|