|
TITLE:
|
ON REFINED AND ROBUST INFERENCES FOR SPATIAL ECONOMETRICS MODELS
|
ABSTRACT
Asymptotically refined and heteroskedasticity robust inferences are considered for spatial linear and panel regression models, based on the quasi maximum likelihood (QML) or the adjusted quasi score (AQS) approaches. Refined inferences are achieved through bias-correcting the QML estimators, bias-correcting the $t$-ratios for covariates effects, and improving tests for spatial effects; heteroskedasticity-robust inferences are achieved through adjusting the quasi score functions. Several popular spatial linear and panel regression models are considered including the linear regression models with either spatial error dependence (SED), or spatial lag dependence (SLD), or both SED and SLD (SARAR), the linear regression models with higher-order spatial effects, SARAR(p,q), and the fixed-effects panel data models with SED or SLD or both. Asymptotic properties of the new estimators and the new inferential statistics are examined.
Chapter 1 introduces in details the issues to be studied in the entire thesis. Chapter 2 focuses on the asymptotic distribution and finite-sample bias correction of the QML Estimators for the linear SED model. Chapter 3 extends Chapter 2 to the linear SARAR model to introduce simple methods for finite-sample bias corrections and for improved t-ratios for covariate and spatial effects. Chapter 4 presents a set of refined inferences methods for the fixed-effects panel data models. Chapter 5 moves onto inferences methods robust against unknown cross-sectional heteroskedasticity, with a focus on the linear SLD model. Chapter 6 introduces a general methodology for heteroskedasticity-robust estimation and inference for all the popular spatial econometric models, with detailed demonstrations given using the linear SARAR(p,q) model, and the fixed-effects panel data models. Chapter 7 concludes the thesis.
|
|
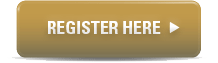
|
PRESENTER
Liu Shew Fan Singapore Management University
|
RESEARCH FIELDS
Spatial Econometrics, Econometrics Theory
|
DATE:
20 May 2016 (Friday)
|
TIME:
10.30am - 12.30pm
|
VENUE:
Seminar Room 3.5, Level 3
School of Economics
Singapore Management University
90 Stamford Road
Singapore 178903
|
|
|
|