|
TOPIC:
|
Infinite Order Cross-Validated Local Polynomial Regression
|
ABSTRACT
Many practical problems require nonparametric estimates of regression functions, and local polynomial regression has emerged as a leading approach. In applied settings practitioners often adopt either the local constant or local linear variants, or choose the order of the local polynomial to be slightly greater than the order of the maximum derivative estimate required. But such ad hoc determination of the polynomial order may not be optimal in general, while the joint determination of the polynomial order and bandwidth presents some interesting theoretical and practical challenges. In this paper we propose a data-driven approach towards the joint determination of the polynomial order and bandwidth, provide theoretical underpinnings, and demonstrate that improvements in both finite-sample efficiency and rates of convergence can thereby be obtained. In the case where the true data generating process (DGP) is in fact a polynomial whose order does not depend on the sample size, our method is capable of attaining the √n rate often associated with correctly specified parametric models, while the estimator is shown to be uniformly consistent for a much larger class of DGPs. Theoretical underpinnings are provided and finite-sample properties are examined.
Please click here to download paper.
|
|
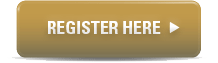
|
Presenter
Jeffrey Scott Racine
McMaster University
|
Research Fields
Nonparametric estimation and inference, cross-validatory model selection, nonparametric instrumental methods, and entropy-based measures of dependence and their statistical underpinnings.
|
Date:
26 February 2014 (Wednesday)
|
Time:
4pm - 5.30pm
|
Venue:
Seminar Room 5.1, Level 5
School of Economics
Singapore Management University
90 Stamford Road
Singapore 178903
|
|
|
|